The Implications of Child Sexual Abuse Material in AI Training Datasets
The Implications of Child Sexual Abuse Material in AI Training Datasets
The use of artificial intelligence (AI) training datasets is a crucial aspect of developing AI systems. However, the presence of child sexual abuse material (CSAM) within these datasets has raised significant ethical and legal concerns. The inclusion of CSAM in AI training datasets can perpetuate the exploitation of children and contribute to the dissemination of illegal content.
The implications of using AI training datasets containing CSAM are multifaceted. Firstly, it poses a serious risk of re-victimization as the images or videos may depict actual instances of child sexual abuse. Moreover, the distribution and use of such material for training AI models can inadvertently contribute to the proliferation of CSAM.
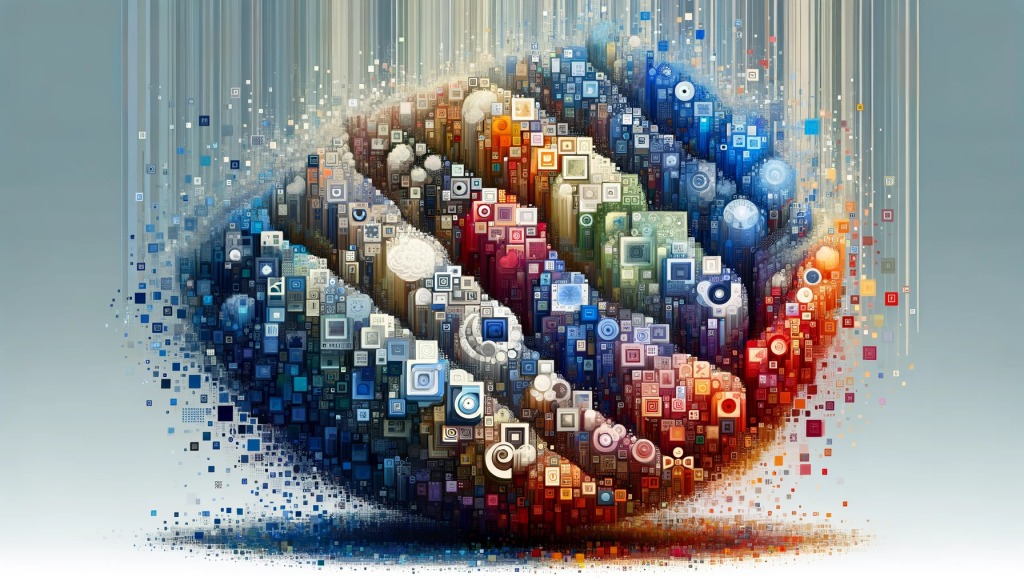
Artificial intelligence (AI) has revolutionized various industries, including image generation. However, a recent study by Stanford Internet Observatory researchers has uncovered a disturbing revelation. They found over a thousand instances of child sexual abuse material in a massive public dataset known as LAION 5B, used to train popular AI image-generating models. This discovery raises concerns about the potential for AI models to create realistic “deepfake” images of child exploitation. Additionally, it highlights the need for transparency and safety in the training data used for generative AI tools.
The LAION 5B Dataset
The LAION 5B dataset, created by the German nonprofit organization LAION, contains billions of images scraped from various sources, including social media and adult entertainment websites. Out of the five billion images in the dataset, the researchers identified at least 1,008 instances of child sexual abuse material. LAION has a “zero tolerance policy for illegal content” and is currently evaluating the findings. The organization also mentioned its collaboration with the UK-based Internet Watch Foundation to identify and remove suspicious and potentially unlawful content from the dataset.
Addressing the Issue
Upon discovering the presence of child sexual abuse material in the LAION 5B dataset, the Stanford researchers took immediate action. They reported the image URLs to the National Center for Missing and Exploited Children and the Canadian Centre for Child Protection for further investigation and removal. LAION, on the other hand, has taken LAION 5B offline temporarily and plans to conduct a full safety review before republishing the dataset.
Filtering and Training Process
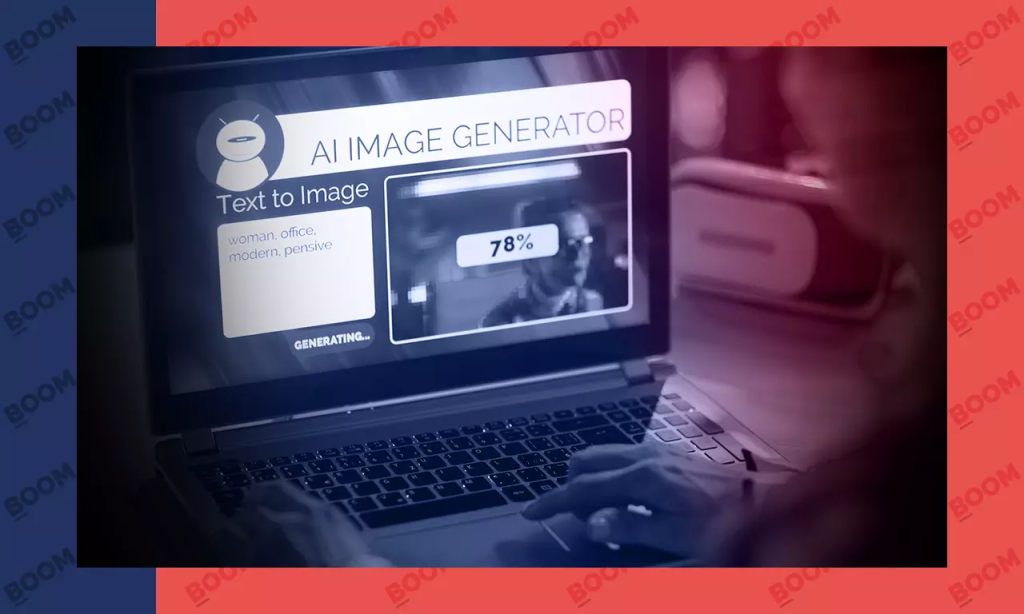
The Stanford researchers noted that LAION attempted to filter explicit content from the dataset. However, an earlier version of the popular image-generating model Stable Diffusion, known as Stable Diffusion 1.5, was trained on a wide array of content, including explicit material. Stability AI, the London-based startup behind Stable Diffusion, clarified that Stable Diffusion 1.5 was released by a separate company, not by Stability AI itself. They emphasized that Stable Diffusion 2.0, which largely filtered out explicit material, is the version used by Stability AI and includes additional filters to prevent the generation of unsafe content.
The Role of Stability AI
Stability AI, committed to preventing the generation of unsafe content, only hosts versions of Stable Diffusion that have filters to remove explicit material. By eliminating such content before it reaches the model, Stability AI aims to mitigate potential risks. The company explicitly prohibits the use of its products for unlawful activities. However, the Stanford researchers noted that Stable Diffusion 1.5, the earlier version still in use in some corners of the internet, remains popular for generating explicit imagery. As part of their recommendations, the researchers suggest deprecating and ceasing the distribution of models based on Stable Diffusion 1.5.
Concerns with Web-Scale Datasets
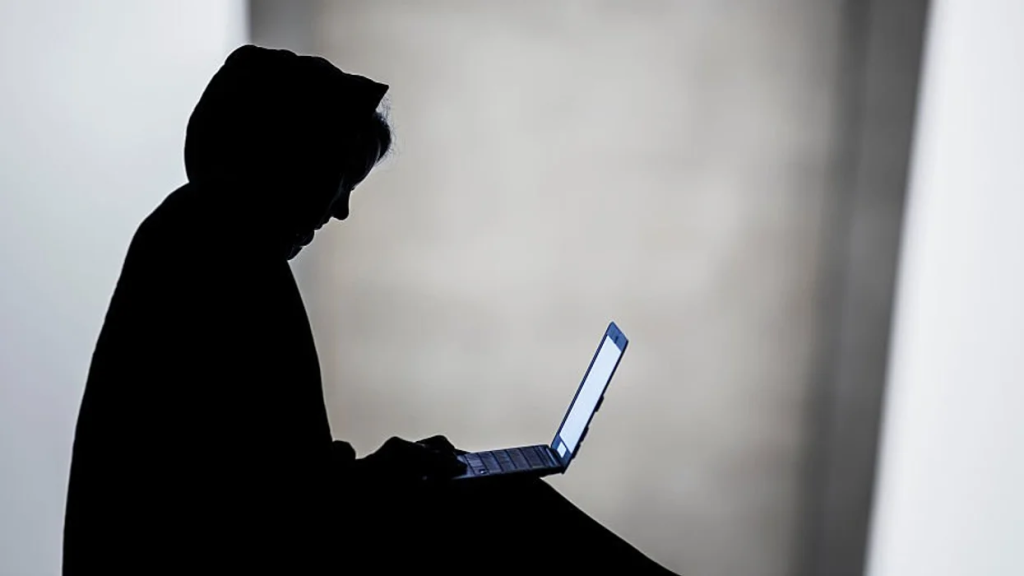
The Stanford report highlights the challenges posed by massive web-scale datasets, not only in terms of child sexual abuse material but also regarding privacy and copyright concerns. Even with safety filtering attempts, these datasets can inadvertently include explicit content. The researchers recommend restricting such datasets to research settings only and using more curated and well-sourced datasets for publicly distributed models.
Conclusion
The discovery of child sexual abuse material in the LAION 5B dataset used to train AI image-generating models raises serious concerns about the potential for AI to create realistic deepfake images of child exploitation. It emphasizes the need for transparency and safety in the training data used for generative AI tools. LAION and Stability AI have taken steps to address the issue, including evaluating the dataset and implementing filters to prevent the generation of explicit content. Moving forward, it is crucial to prioritize the use of curated and well-sourced datasets to ensure the ethical and responsible use of AI technology. By doing so, we can mitigate the risks associated with AI-generated content and protect vulnerable individuals from harm.